👋 Hi there!
I am a PhD candidate at the University of Southern California's (USC) Information Sciences Institute (ISI), advised by Jonathan May.
My research is in natural language processing and machine learning, and most of my research have been centered around improving AI models through enhanced contextualization and analyzing their role in human-AI interactions. I've always been mesmerized by likeable and superintelligent digital assistants in science fiction such as Samantha in Her and TARS in Interstellar, and I'm excited to make them a reality.
Previously, I did my undergraduate studies at Hong Kong University of Science and Technology with a major in Computer Science, where I first worked on NLP with Pascale Fung. I have also interned at Amazon Alexa (now Amazon AGI), working with Nicolaas Jedema, Alessandro Moschitti, and Pedro Szekely (2023, 2024), at Meta AI on Alborz Geramifard's Cognitive AI team with Chinnadhurai Sankar (2022) and with Ahmad Beirami (2021), Stitch Fix (2020), and at ISI's Natural Language Group with Jonathan May (2019).
📆 Office hours
I host virtual office hours! I've had the pleasure making many new connections through these office hours. I'm open to discussing anything from research to career advice that I can help with.
Please schedule through Calendly.
Email me if the listed time slots don't work for you.
🔍 Research
Check google scholar for my full publication list.
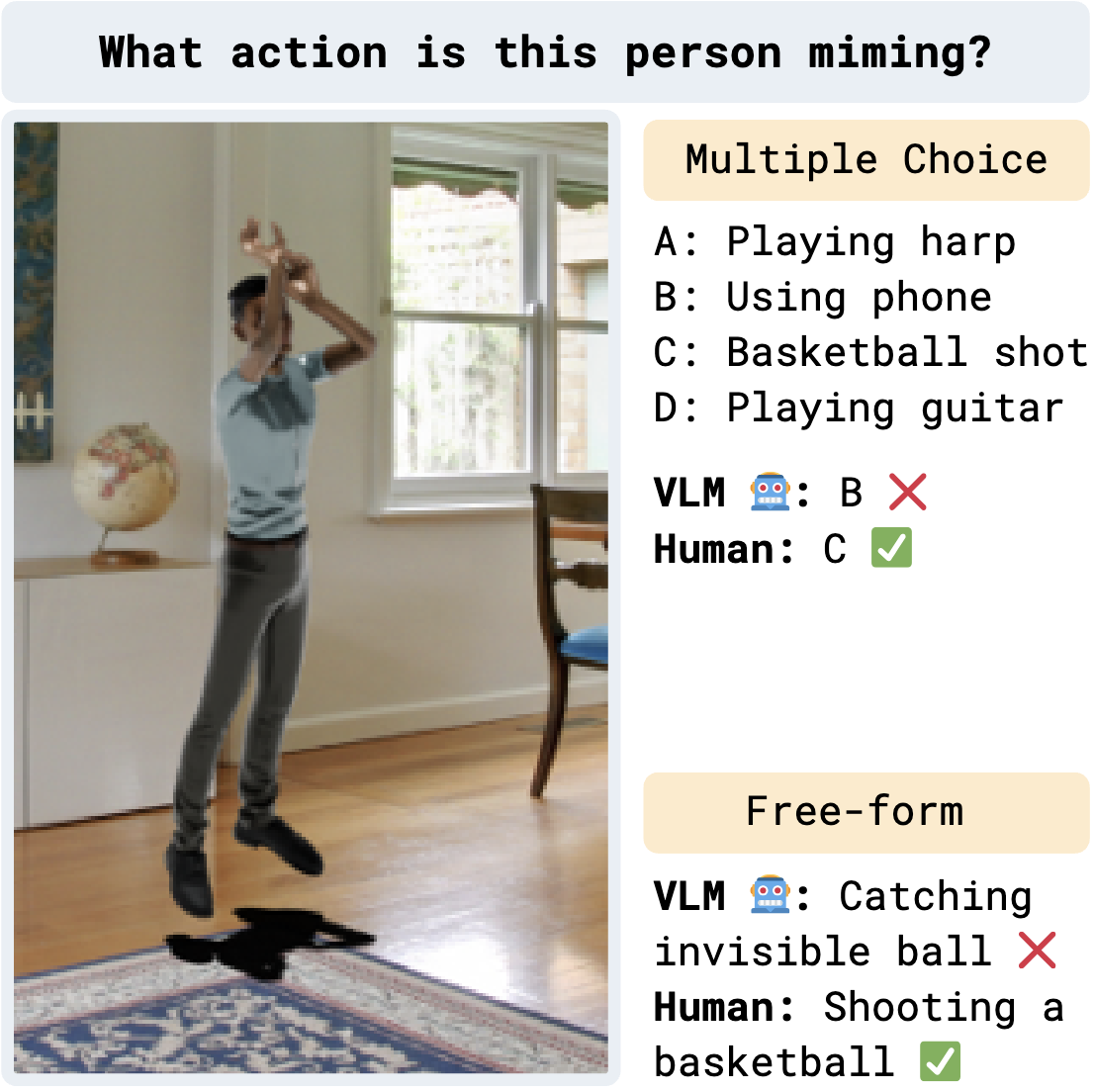
We propose Mime Identification Multimodal Evaluation (MIME), a novel video-based question answering benchmark comprising of 86 mimed actions, as a step towards understanding nonverbal communication.
We find that both open-weight and API-based vision-language models perform significantly worse than humans on MIME, motivating the need for increased research for instilling more robust understanding of human gestures.
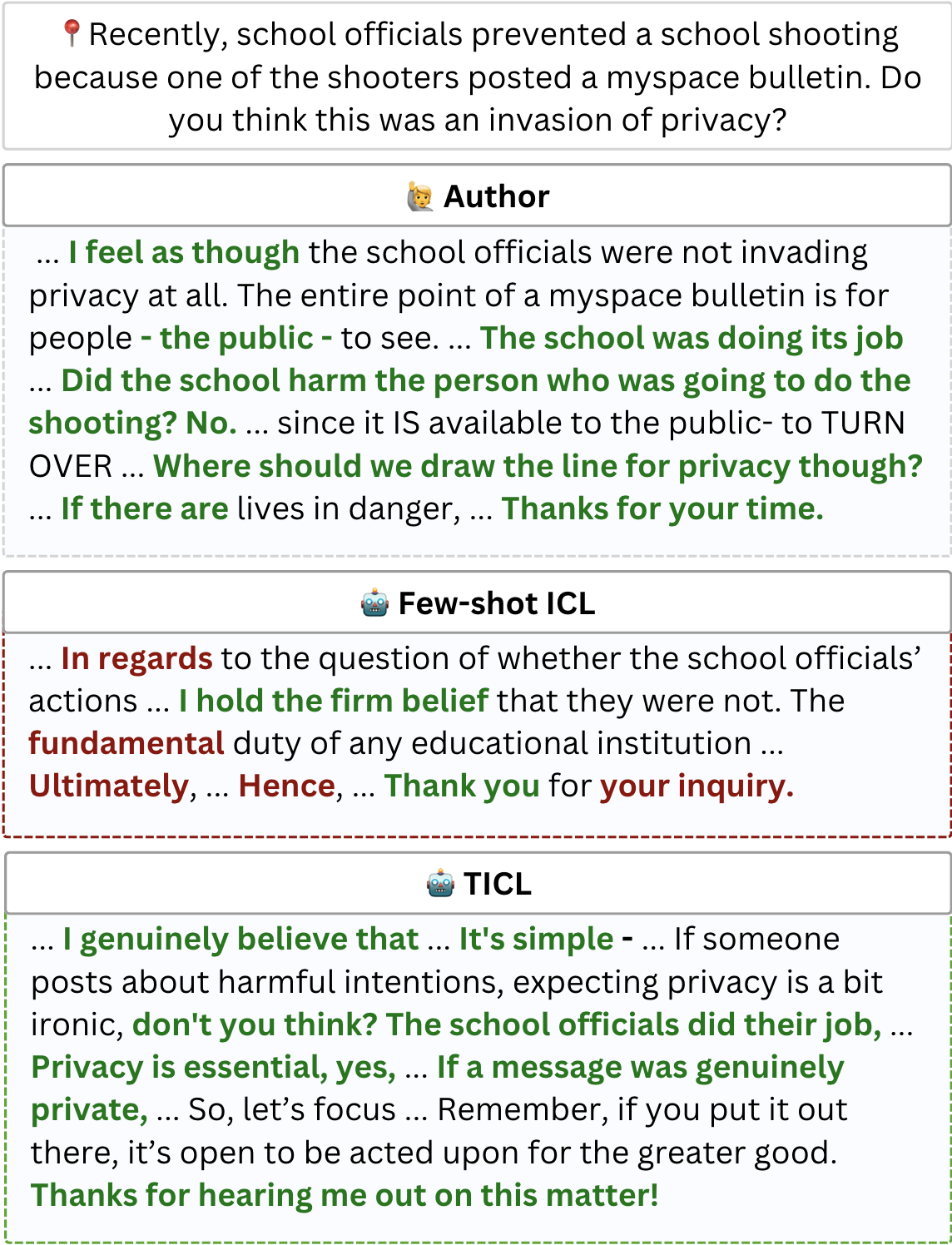
We present a tuning-free method that personalizes language models for text generation tasks with fewer than 10 examples per user. TICL iteratively expands an in-context learning prompt via a trial-error-explain process, adding model-generated negative samples and explanations that provide fine-grained guidance towards a specific user's style. We show that the negative samples and explanations enable language models to learn stylistic context more effectively and overcome the bias towards structural and formal phrases observed in their zero-shot outputs and few-shot outputs.
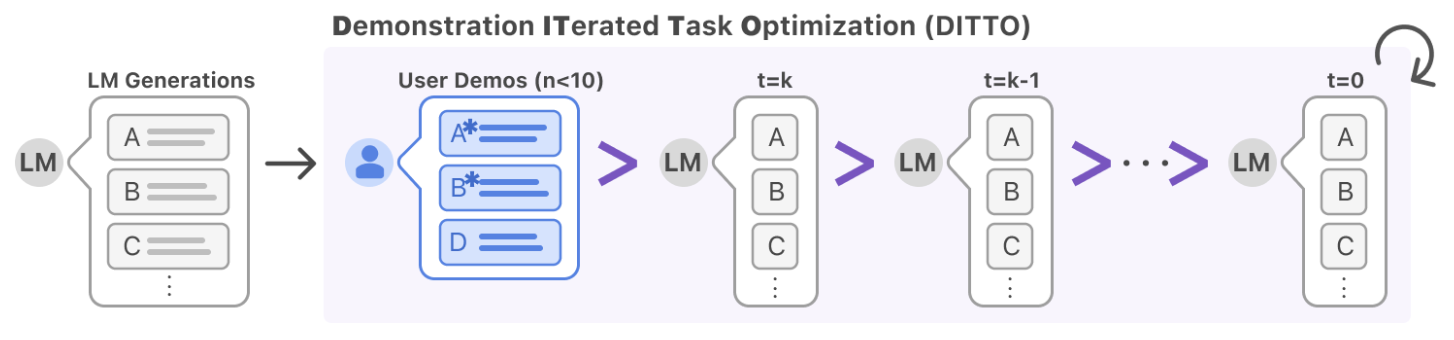
We show that it is possible to align an LLM to a specific setting by leveraging a very small number (<10) of demonstrations as feedback. Our method, Demonstration ITerated Task Optimization (DITTO), directly aligns language model outputs to a user's demonstrated behaviors. Derived using ideas from online imitation learning, DITTO cheaply generates online comparison data by treating users' demonstrations as preferred over output from the LLM and its intermediate checkpoints. Across our benchmarks and user study, we find that win-rates for DITTO outperform few-shot prompting, supervised fine-tuning, and other self-play methods by an average of 19% points. By using demonstrations as feedback directly, DITTO offers a novel method for effective customization of LLMs.
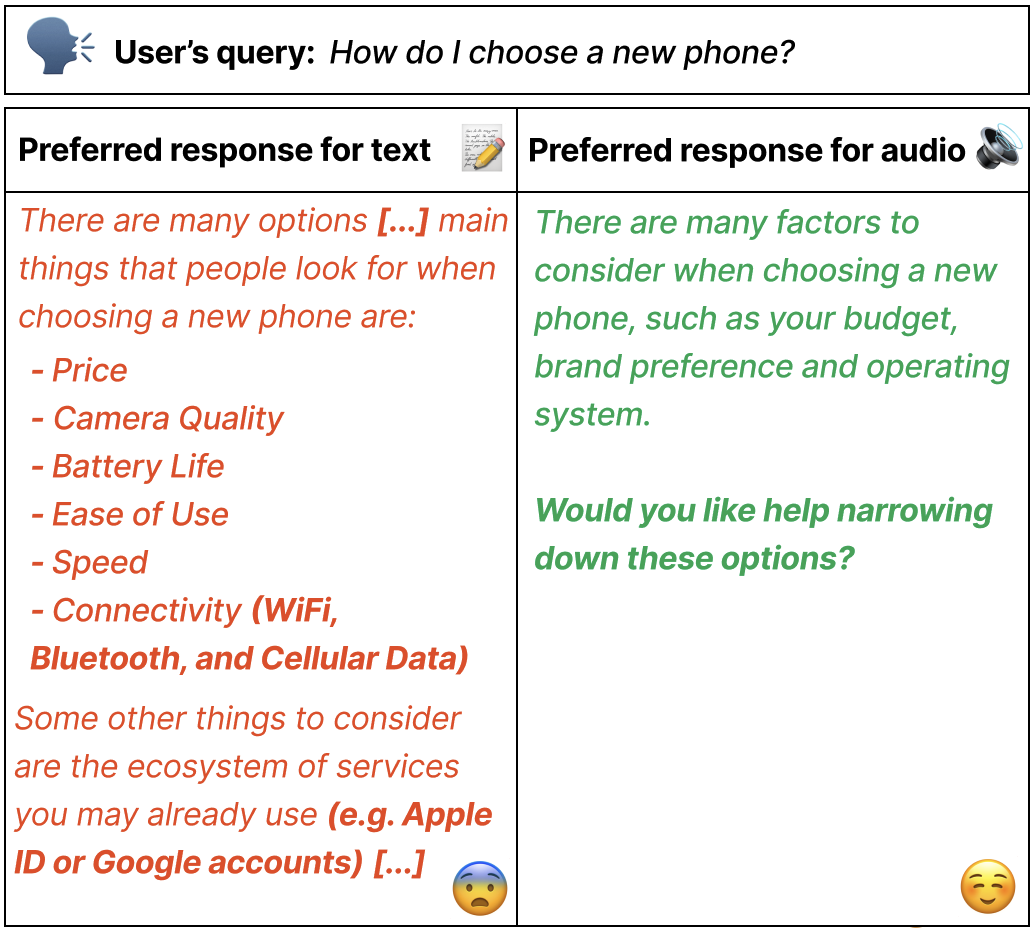
Current LLMs are fine-tuned with data exclusively with a text interface, which does not capture human preferences for speech, and thus generate text that is not suitable for text-to-speech systems.
We collect 20K human preference data where annotators listen to the paired responses, instead of reading them through a text interface. We use this data for reinforcement learning with human feedback to adapt an instruction-tuned language model to generate speech-suitable text.
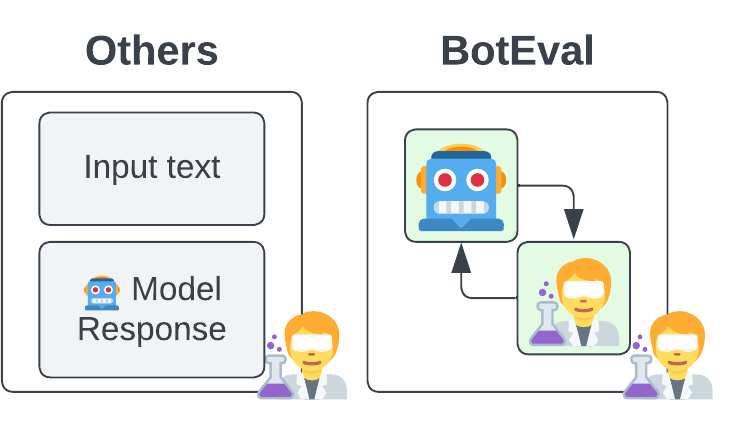
We develop BotEval, an easily customizable, open-source, evaluation toolkit that focuses on enabling human-bot interactions as part of the evaluation process, as opposed to human evaluators evaluating a static input.
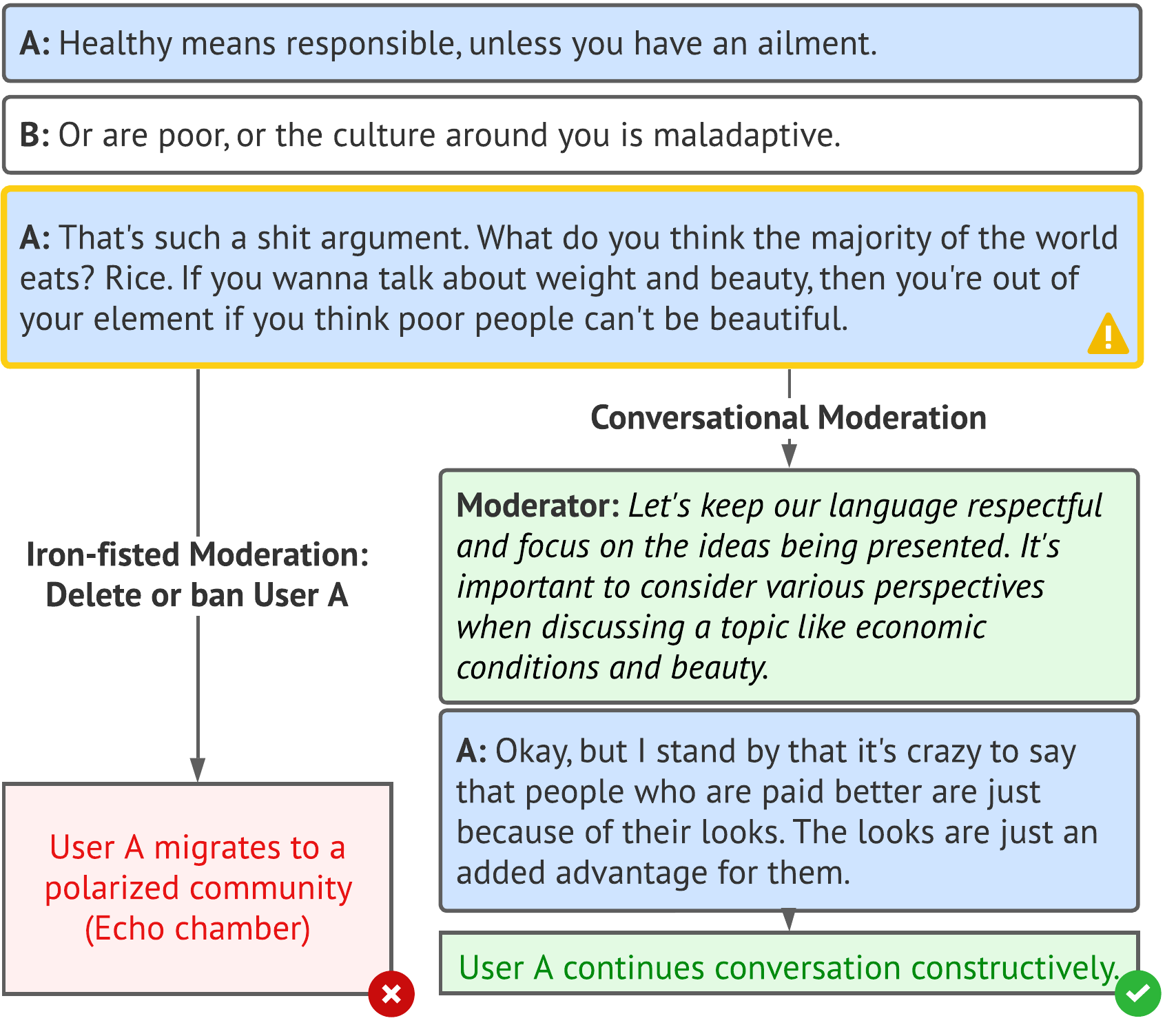
Deleting comments and banning users are iron-fisted moderation tactics that can lead to a chilling effect on free speech. Instead, conversational moderation aims to guide users to more constructive behavior.
We investigate whether language models can be effective conversational moderators and thus be effective tools for improving the health of online discourse.
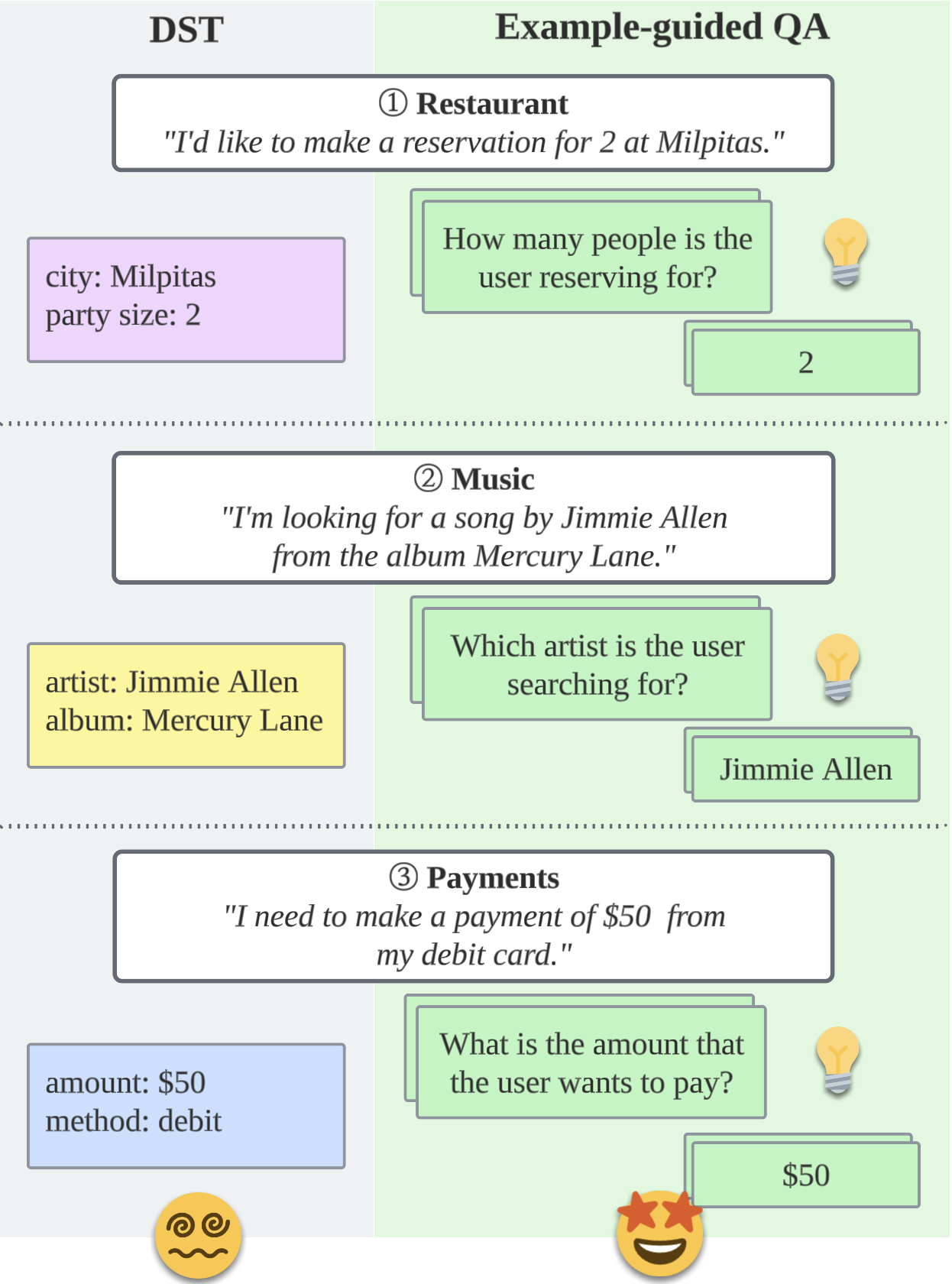
Estimating a user's goal in a dialogue can be done by asking natural language questions, and answering questions is a transferable skill that can be easily learned from examples.
With this insight, we restructure dialogue state tracking (DST) to eliminate service-specific structured text and unify data from all services by decomposing each DST sample to a bundle of fine-grained example-guided question answering tasks. With a retriever trained to find examples that introduce similar updates to dialogue states, we find that our method can significantly boost continual learning performance, even for a model with just 60M parameters.
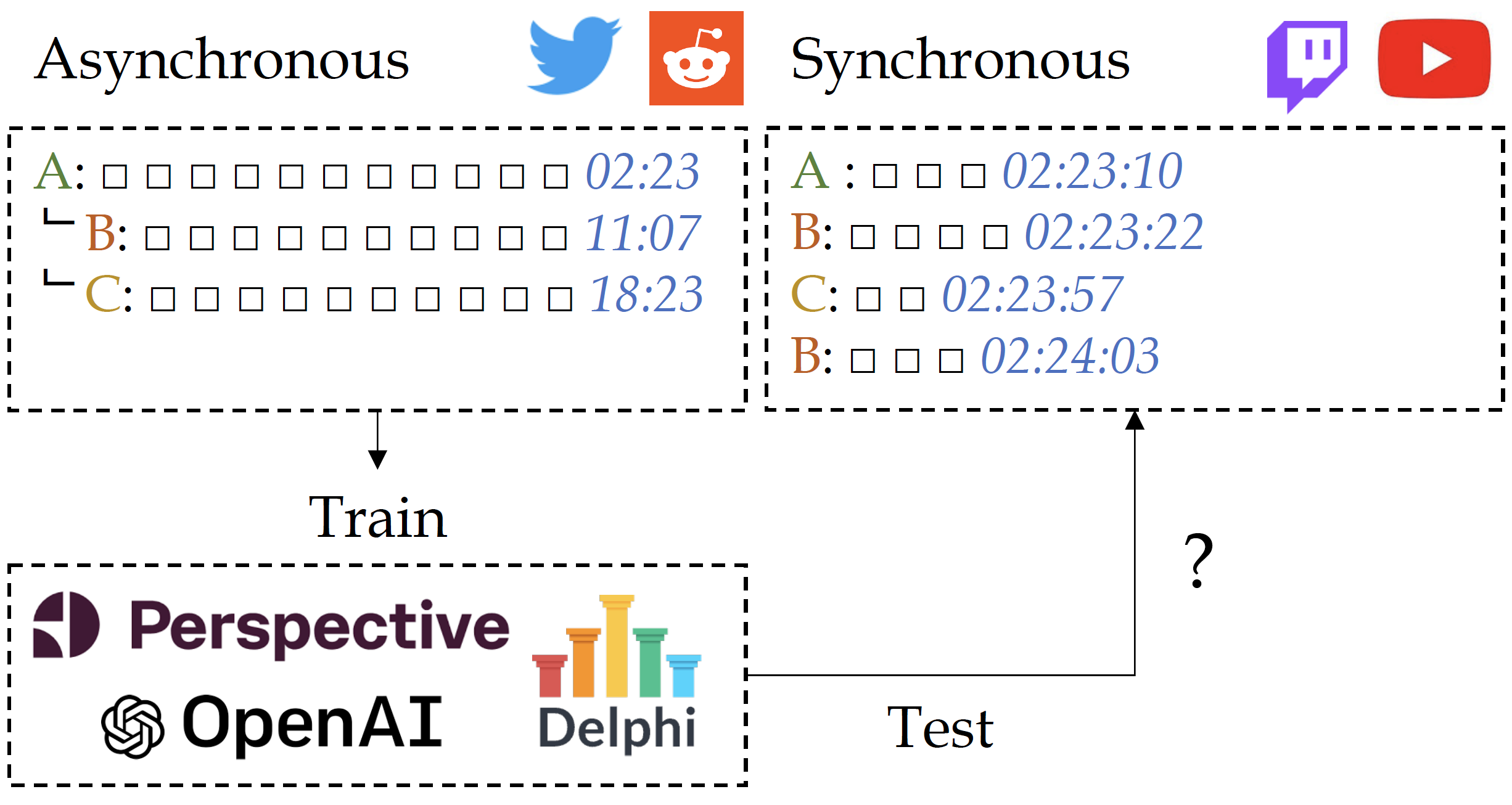
Toxic behavior in live-stream chat is a growing concern as live-streaming platforms such as Twitch and YouTube live are becoming increasingly popular.
Previous detection methods are not effective for live-stream chat as each comment is only visible for a limited time and lacks a thread structure. To bridge this gap, we define norm violation categories in live-stream chats and annotate 4,583 moderated comments from Twitch and train live-stream chat-specific detection models.
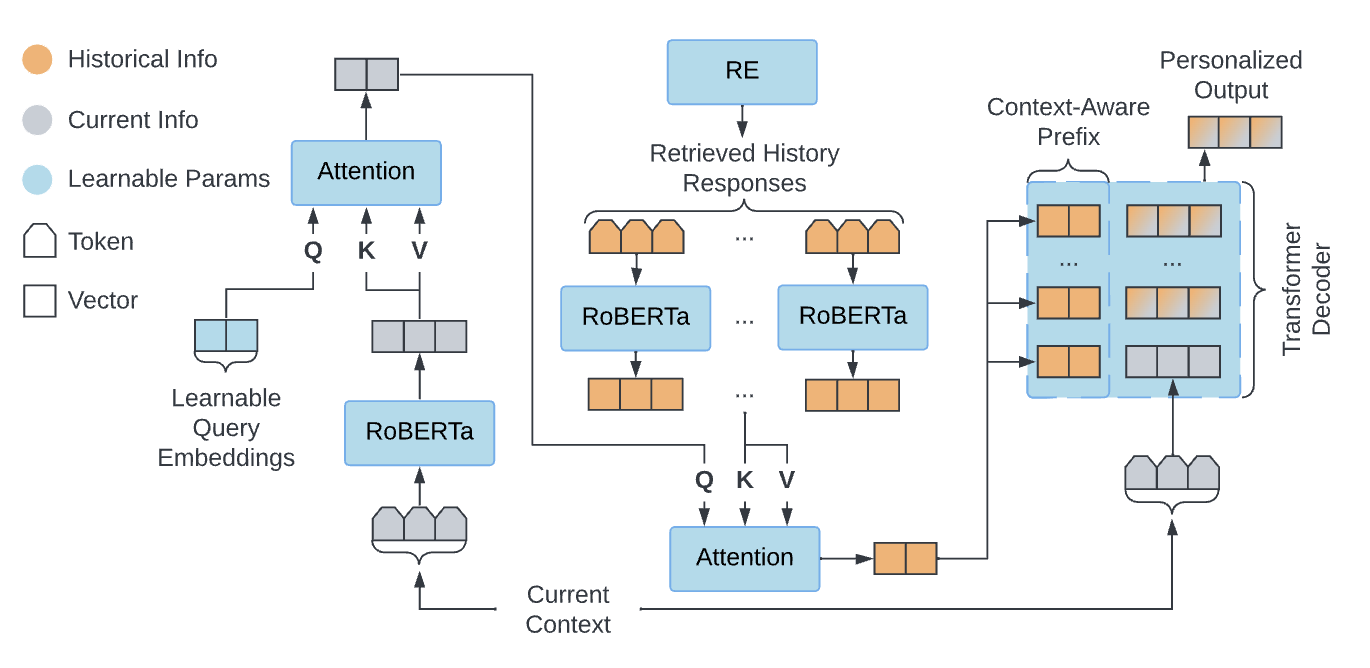
RECAP: Retrieval-Enhanced Context-Aware Prefix Encoder for Personalized Dialogue Response Generation
Endowing chatbots with a consistent persona is essential to an engaging conversation, yet it remains an unresolved challenge. In this work, we propose a new retrieval-enhanced approach for personalized response generation.
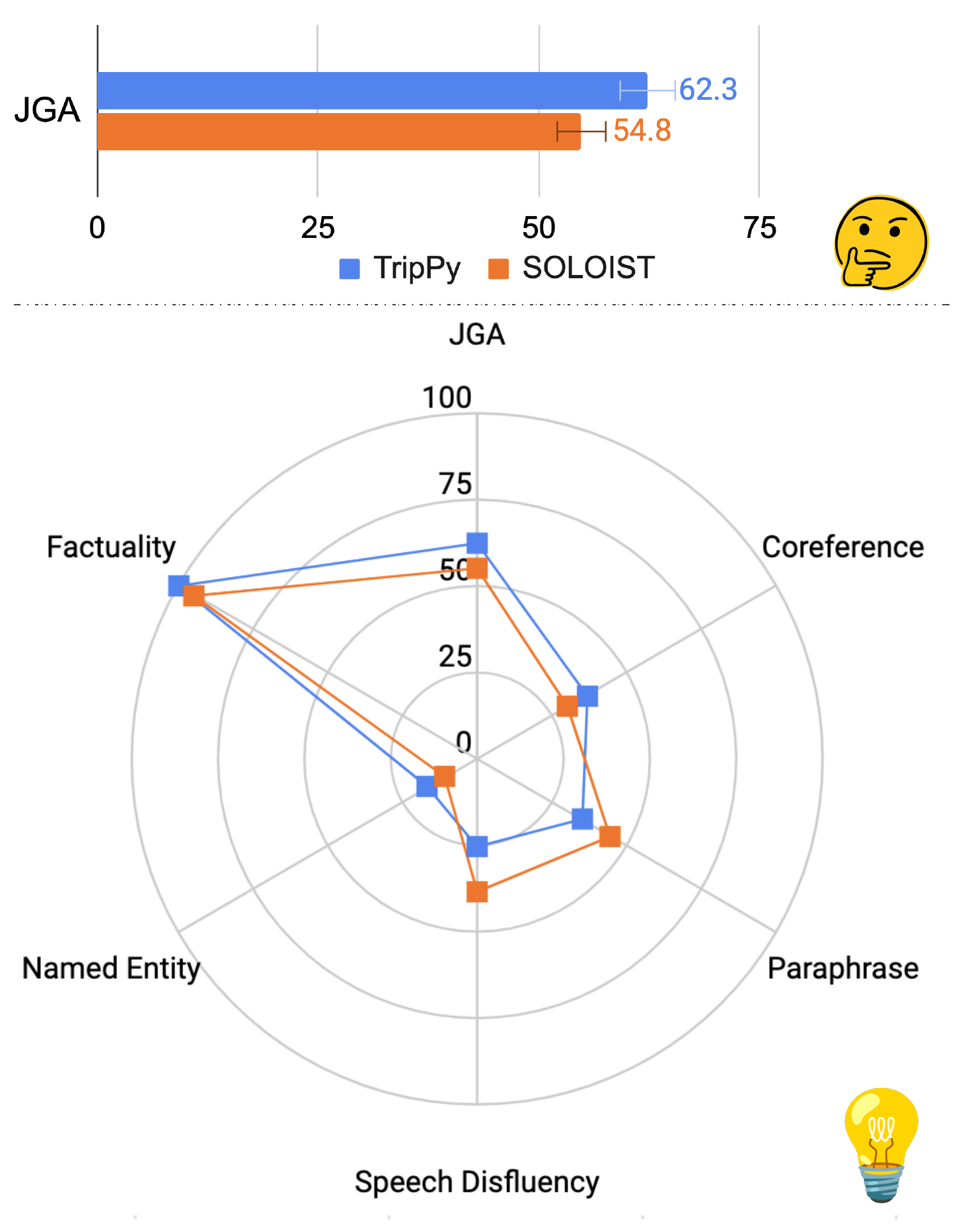
Humans are robust to understanding dialogue states in the presence of noise and ambiguity, but dialogue state tracking (DST) models are not. This analysis of DST robustness has been sparse and uncoordinated in previous work. Our standardized and comprehensive DST diagnoses toolkit, CheckDST, is a collection of robustness tests and failure mode analytics. With CheckDST, we discover that different classes of DST models have clear strengths and weaknesses, where generation models are more promising for handling language variety while classification models are more robust to unseen entities.
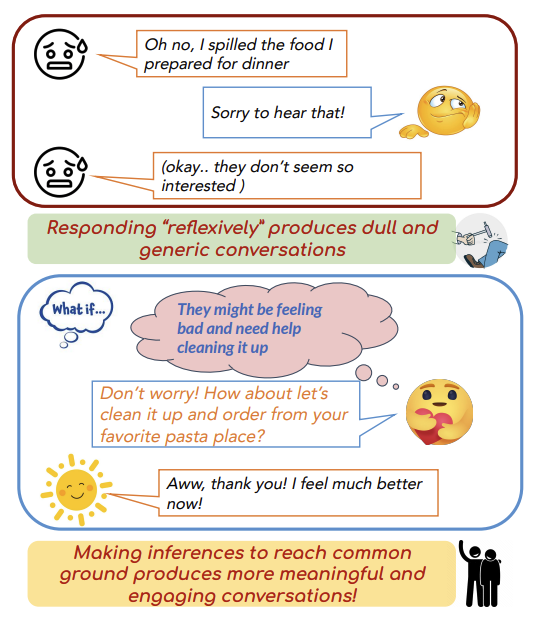
We introduce Reflect, a dataset that annotates dialogues with explicit CG and solicits 9k diverse human-generated responses each following one common ground. Using Reflect, we showcase the limitations of current dialogue data and RG models: less than half of the responses in current data are rated as high quality and models trained using this data have even lower quality, while most Reflect responses are judged high quality. We also analyze whether CG can help models produce better-quality responses by using Reflect CG to guide RG models.
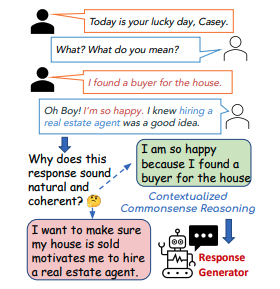
We collect 6k annotated explanations justifying responses from four dialogue datasets and ask humans to verify them and propose two probing settings to evaluate response generation models' commonsense reasoning capabilities. Probing results show that response generation models fail to capture the logical relations between commonsense explanations and responses and fine-tuning on in-domain data and increasing model sizes do not lead to understanding of commonsense reasoning.
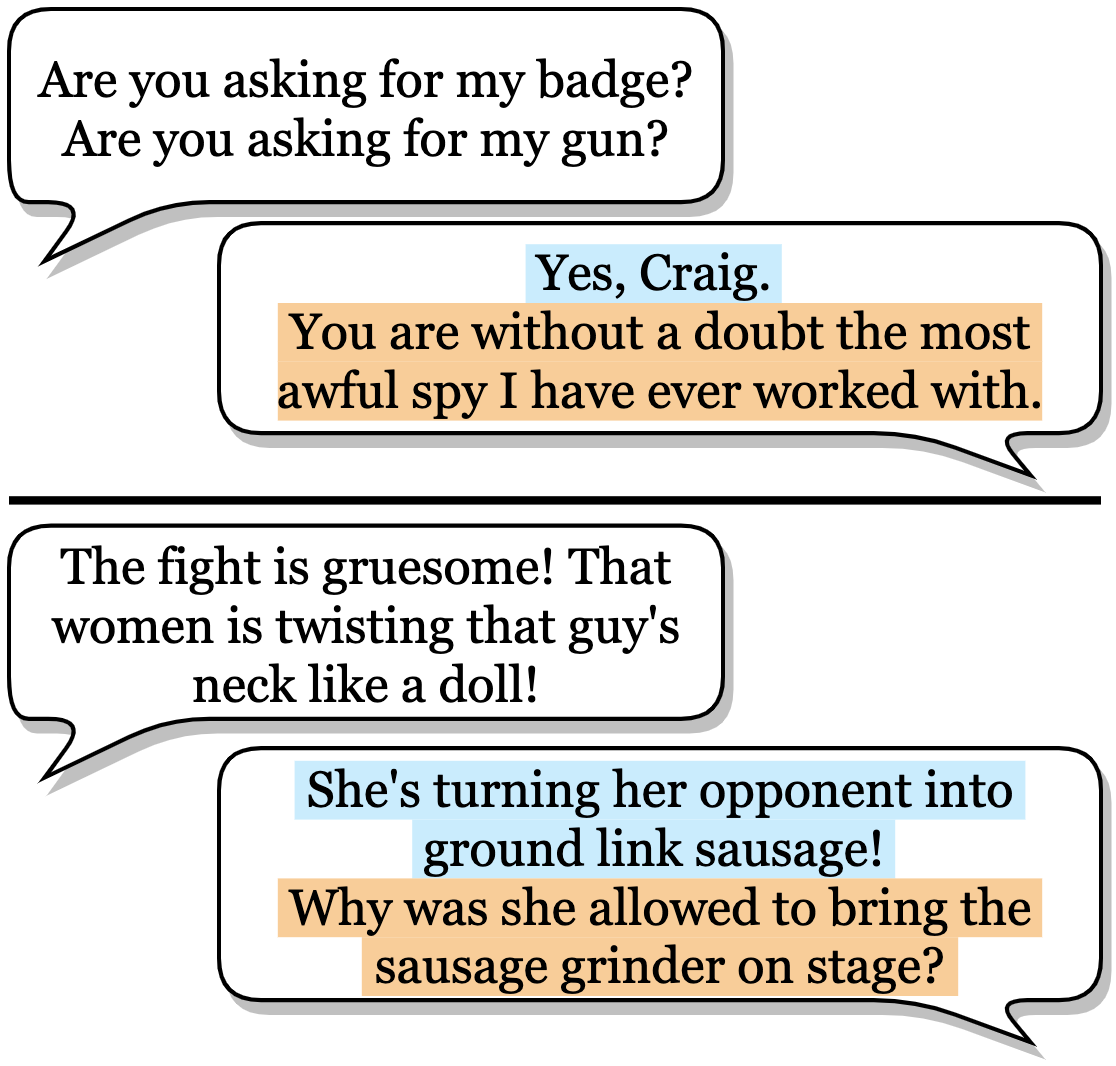
Open-domain dialogue systems overlook an important phenomena that makes conversations engaging: the initiation of the next relevant contribution, which is the most proactive method of "grounding". We collect "Yes, and" type dialogue pairs that naturally embed such initations from an improv podcast and existing dialogue corpora to create the Spontaneanation Pairs Of Learnable ImprovisatioN (SPOLIN) dataset. Human evaluation shows that models fine-tuned with SPOLIN generate more engaging results.
🔍 Preprints / Others
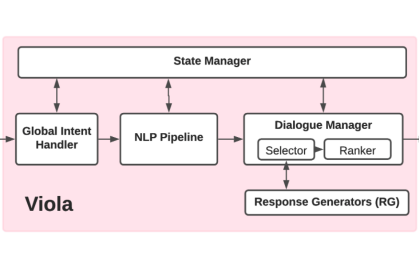
We present Viola, an open-domain dialogue system based on a simple generate-and-rank approach. Viola fetches a batch of response candidates from various neural dialogue models and template-based generators and chooses the final response with a poly-encoder ranker fine-tuned with annotated Alexa conversation data.
📢 News
- 2025/5: "Can Vision-Language Models Understand Mimed Actions?" (Findings) and "NewsInterview: a Dataset and a Playground to Evaluate LLMs' Ground Gap via Informational Interviews!" (Main) have been accepted to ACL 2025!
- 2025/1: "Tuning-Free Personalized Alignment via Trial-Error-Explain In-Context Learning" has been accepted to NAACL 2025 Findings! "Aligning Language Models with Demonstrated Feedback" has been accepted to ICLR 2025!
- 2024/9: Our work "Speechworthy Instruction-tuned Language Models" has been accepted to EMNLP 2024! See you in Miami!
- 2024/6: Our work "BotEval: Facilitating Interactive Human Evaluation" has been accepted to ACL 2024 SDT! I've also started my internship with Amazon as an applied scientist intern.
- 2024/3: Our work "Can Language Model Moderators Improve the Health of Online Discourse?" has been accepted to NAACL 2024! See you in Mexico City!
- 2024/2: I'm giving a talk at Tonal on "Large Language Models: The Recipes and Applications of ChatGPT's Engine" to give an overview of recent advances in NLP and my work on Speechworthy Instruction-tuned Language Models.
- 2023/11: I'll be attending EMNLP 2023 in person to present Continual Dialogue State Tracking via Example-Guided Question Answering and Analyzing Norm Violations in Live-Stream Chat.
- 2023/7: I'm an organizer for ICML 2023's What's left to TEACH chatbots? workshop. See you in Hawaii!
- 2023/6: I've started my internship with Amazon Alexa AI to work on adapting language models for voice-based interactions using reinforcement learning with human feedback.
- 2022/11: I'll be attending EMNLP 2022 in person to present Know Thy Strengths: Comprehensive Dialogue State Tracking Diagnostics and Reflect, Not Reflex: Inference-Based Common Ground Improves Dialogue Response Quality.
- 2022/6: I've started my summer internship at Meta AI to work on continual learning.
- 2021/8: I started my internship with Meta AI's Conversational AI team to work on the robustness of task-oriented dialogue models.
- 2021/8: "Probing Causal Common Sense in Dialogue Response Generation", work with Pei Zhou, has been accepted to EMNLP2021 Findings.
- 2021/4: Viola makes it to the semi-finals of the Alexa Prize Socialbot Grand Challenge 4!
- 2020/11: Viola is one of the teams accepted to compete in the Alexa Prize Socialbot Grand Challenge 4! I will be leading the team with Jonathan May as our faculty advisor.
- 2020/9: Stitch Fix posted a blog post about my internship project using NLP to process client feedback for its products. Check it out!
- 2020/9: USC Viterbi Magazine covered Jonathan May's and my SPOLIN work that was published at ACL2020, with a fun video demonstration featuring Mike Henry (Family Guy, The Orville). Check it out!
- 2020/8: I am starting my first semester as a PhD student at USC.
- 2020/6: I will be working at Stitch Fix as a data science intern in the merch product development team.
- 2020/4: My paper with Jonathan May has been accepted to ACL2020! I will be presenting virtually at the conference.
➕ Miscellaneous
- My pronouns are he, him, his.
- Thanks to my dad being a diplomat, I have been blessed with growing up in many different countries: Moscow, Russia; Oslo, Norway; Vienna, Austria; Abu Dhabi, UAE; Hong Kong; Tehran, Iran; Seoul, South Korea. Unfortunately, I haven't lived long enough in most of these countries to master a new language other than Korean and English. My favorite country to live in was Austria, where I spent 5-7th grade. I loved being in central Europe, which was convenient for traveling to many nearby countries.
- I'm a bit of a productivity geek. Ask me about knowledge management and productivity tips! I use Logseq for knowledge management and use tips from How to Take Smart Notes, which recommends the Zettelkasten method. I use Brick for making my phone a dumb phone and Screentime and Jomo for blocking distractions on my laptop while working.
- I love playing football⚽ and I am a huge fan of FC Barcelona. Visca el Barça!
- I am a citizen of South Korea and the US. I am fluent in both English and Korean. I am casually learning Mandarin. 我希望能在不远的将来用流利的中文和你交流。